AI in Business Intelligence & Data Analytics - What it really means
Author
Jake FriedenbergDate Published
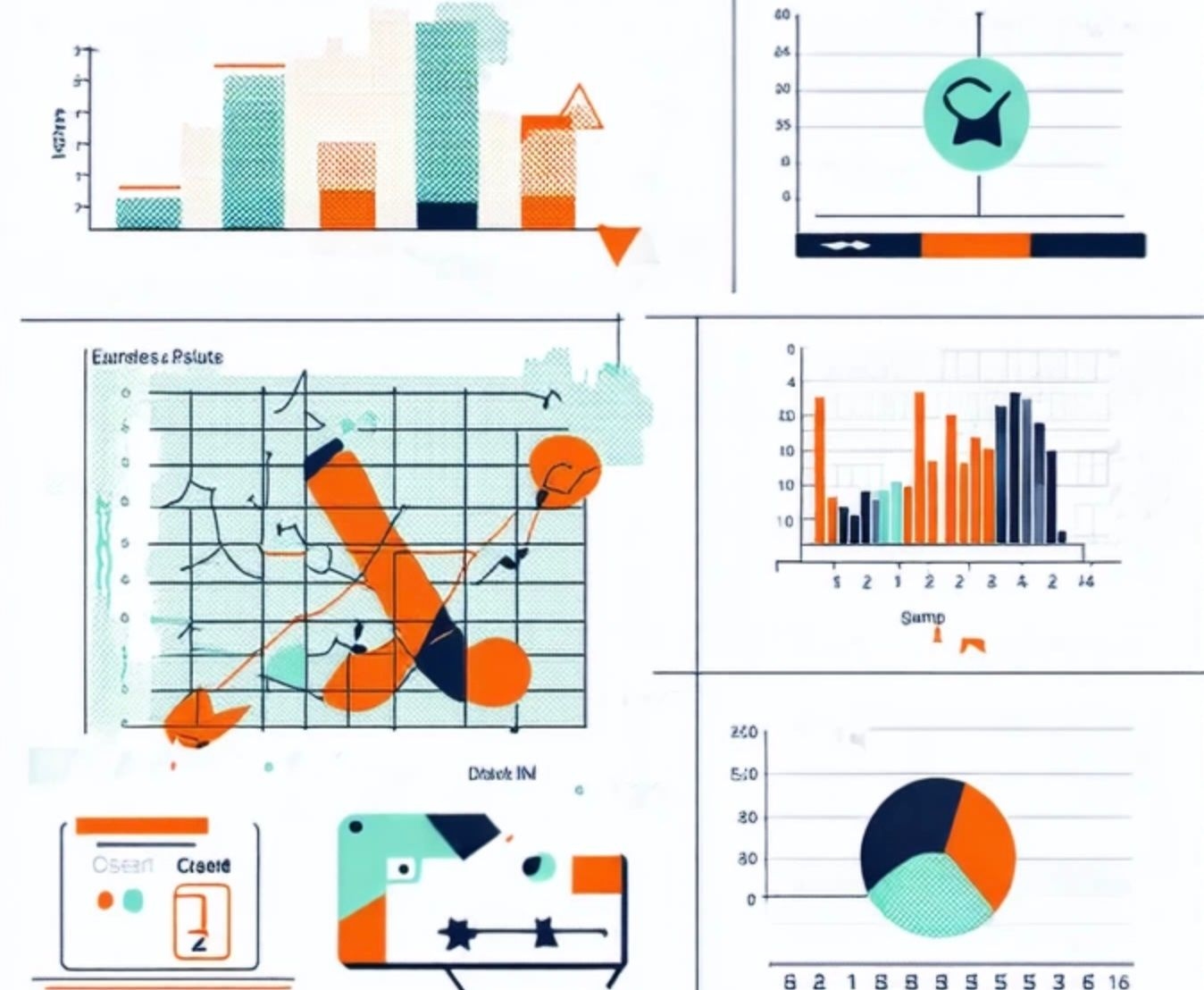
The difference between business intelligence and data analytics has long been a point of discussion in enterprise technology. Traditional BI excels at reporting and visualization, while data analytics provides deeper insights through statistical analysis and machine learning. Yet both approaches share a critical limitation: they tell you what happened or what might happen, but leave you to figure out what to do about it.
When comparing BI vs analytics capabilities, this gap becomes clear. Business intelligence dashboards show what happened, and big data analytics predicts what might happen next. But neither directly bridges the gap between insight and action. This is where autonomous analytics platforms are transforming the enterprise decision-making landscape.
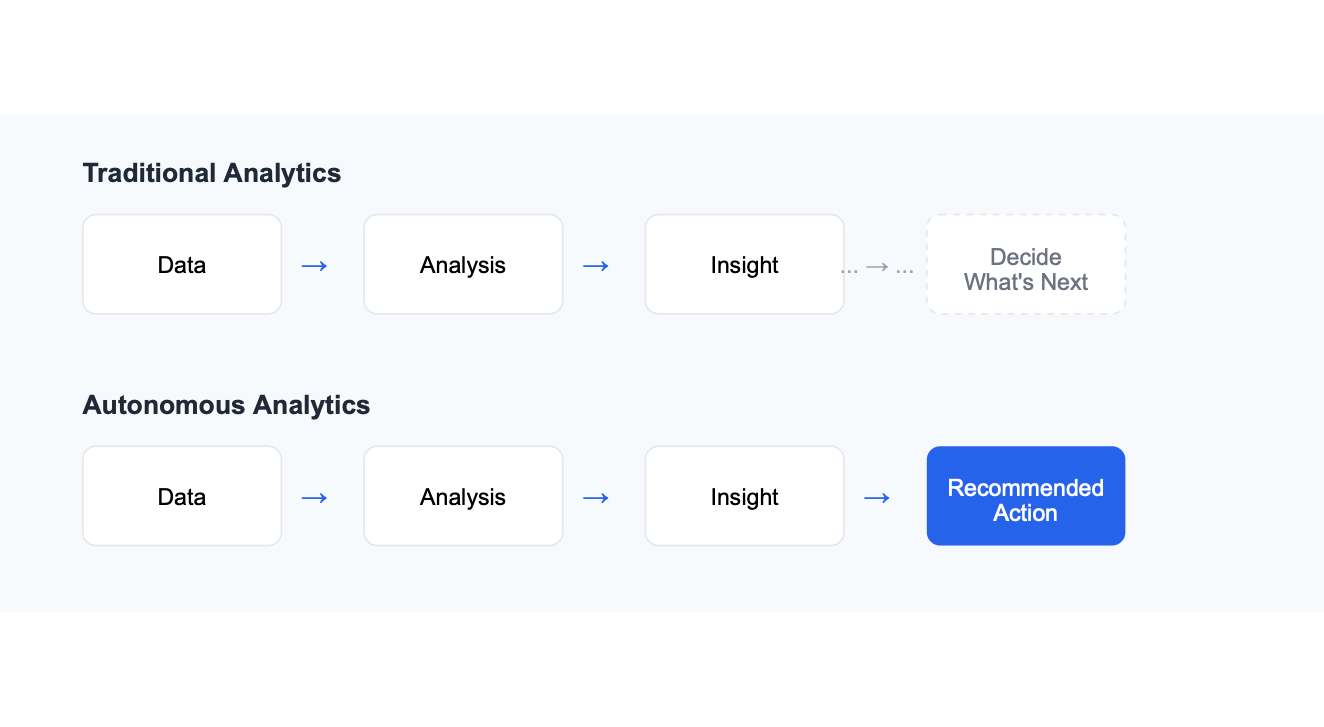
Traditional analytics leaves teams to determine next steps from insights, while autonomous analytics provides specific recommended actions.
Customer Success Operations
Traditional Business Intelligence & Data Analytics: Your BI dashboard shows increasing churn rates. Your big data analytics team builds a predictive model showing 85% accuracy in identifying at-risk customers. Your analysts spend weeks analyzing these insights to develop intervention strategies.
Autonomous Analytics: The system automatically correlates customer behavior, support data, and product usage patterns to identify that support delays during APAC hours drive 60% of churn risk. Based on historical success patterns and current resource constraints, it recommends specific schedule adjustments projected to prevent 82% of high-risk cases, with an estimated $2.8M revenue impact. These recommendations include:
- Optimal support shift timing for APAC coverage
- Priority routing rules based on customer lifecycle stage
- Resource allocation plans that maintain EMEA/US coverage while addressing APAC gaps
Revenue Operations
Traditional Business Intelligence & Data Analytics: Your BI vs big data analytics tools show different pieces of the puzzle: BI dashboards reveal marketing performance down 15%, while advanced analytics show deal cycles extended by 20 days. Different teams analyze these metrics separately, missing crucial connections.
Autonomous Analytics: The system identifies that recent UI changes are causing confusion in technical evaluations, creating a cascade effect:
- Extended product evaluation periods directly impact deal velocity
- Increased support queries reduce sales engineering availability
- Marketing messages misalign with actual user experience
Based on this analysis, it projects that implementing its recommended UI and message changes would accelerate pipeline velocity by 24% within 30 days. Each recommendation includes projected revenue impact and implementation complexity scores.
Supply Chain Intelligence
Traditional Business Intelligence & Data Analytics: Your business intelligence dashboards flag inventory 20% above target. Big data analytics predict seasonal demand variations. Teams manually piece together optimization strategies.
Autonomous Analytics: The system analyzes patterns across inventory costs, supplier performance, and demand fluctuations, recommending specific adjustments to ordering patterns. It projects $1.2M in annual savings while maintaining 99.8% service levels, based on:
- Historical supplier delivery patterns
- Seasonal demand variations
- Carrying cost analysis
- Current market conditions
The Evolution of Enterprise Analytics
The convergence of business intelligence and data analytics through autonomous systems represents more than technological advancement. It transforms how organizations leverage data:
Business Intelligence Analysts shift from building reports to validating and refining system recommendations.
Data Analysts evolve from processing information to setting optimization parameters and business rules.
Digital Analytics Teams focus on strategic initiatives rather than routine analysis.
This evolution moves beyond the traditional difference between BI and data analytics, creating a new paradigm where systems don't just provide insights but recommend specific actions. Organizations can move from asking "what happened?" to automatically receiving answers to "what should we do next?"
This transformation doesn't eliminate the need for human expertise - instead, it elevates analysts from data processors to strategic advisors, focusing their efforts on validating and implementing system recommendations rather than discovering basic insights.