Enterprise Analytics: The Shift to Autonomous Analytics
Author
Jake FriedenbergDate Published
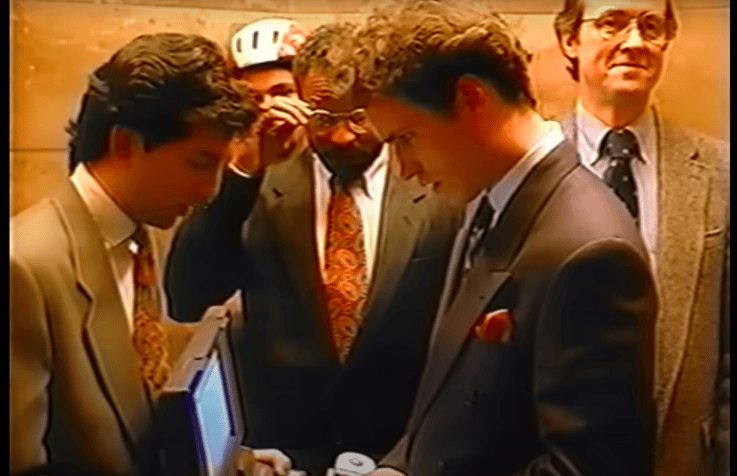
Enterprise analytics is stuck.
Sure, we can now query our data with natural language, or quickly generate good looking graphs at lightning speed. But the fundamentals - a really good source of truth on where you are - is fundamentally just better versions of the same thing.
While companies invest millions in business intelligence automation, most analytics teams are playing catch-up - building dashboards that tell you where you are (if you're lucky), instead of helping you shape what comes next. It's like having a really expensive rearview mirror. Obviously, we all want good rearview mirrors - but don't you also want a GPS system? Think Google Maps for your business: proactively alerting you to problems, suggesting optimal paths, and continuously adapting as conditions change.
There's a shift happening in the way businesses not only derive insights, but determine how to act on them. A new category of autonomous analytics platforms, led by companies like Perceptura, is changing how enterprises think about data. Instead of just visualizing data or automating model building, self-learning analytics platforms actively work to understand your business and drive outcomes.
This autonomous business intelligence represents the next evolution in analytics process automation - what we sometimes call agentic analytics (though you might hear terms like proactive analytics or strategic analytics platforms - more on that later).
The Evolution of Enterprise Analytics Automation
Enterprise analytics has already gone through several major phases. Let's talk about how we got here:
The Spreadsheet Era (1980s - Early 2000s)
Remember when Excel was the height of business intelligence? Companies built entire analytical frameworks on spreadsheets, passing them around like digital hot potatoes. It worked (kind of), but it was manual, error-prone, and about as scalable as a paper airplane.
The Business Intelligence Revolution (2000s - Early 2010s)
Then came the BI tools - suddenly everyone could build pretty dashboards. Data visualization became the name of the game, and for a while, it seemed like we'd solved the problem. But we quickly realized that having beautiful business intelligence dashboards doesn't automatically mean making better decisions.
The Predictive Analytics Wave (2010s - Early 2020s)
“Just add AI” - Predictive business analytics and machine learning. Companies hired armies of data scientists, built complex models, and... often ended up with sophisticated solutions looking for problems to solve. The gap between technical capability and business value remained.
The Autonomous Analytics Future (Hey, how’s it going?)
This is what’s coming in hot and fast. The emergence of autonomous analytics platforms that don't just process data but actually understand your business context and drive outcomes through AI-powered analytics.
Yesterday's Analytics Landscape: Limited To What We Define
The tools we have today are incredibly powerful - they're just not autonomous. The current landscape breaks down into three main categories, each with their own strengths and fundamental limitations:
Visualization Tools
And of course, we have the visualization standbys: Tableau and Power BI. They make beautiful dashboards without breaking a sweat, and even your CEO can figure out how to use them. But they're fundamentally reactive - still just a fancy way to look backward. About as proactive as a teenager on Monday morning, and just as resource-hungry.
Machine Learning Platforms
Then there's DataRobot, the OG of automated machine learning. They've democratized predictive analytics and support pretty much any algorithm you can think of. But it's still stuck in data science land - you need serious expertise to use it effectively, and it's more focused on building models than driving business outcomes. It's like having a rocket ship to go grocery shopping.
Enterprise Data Platforms
The 800-pound gorilla of enterprise data integration would likely be Palantir Foundry. It's a beast at handling complex data and unstructured information, with customization options that would make any developer drool. But it's like bringing a tank to a street race - probably overkill unless you're the Department of Defense, and you'll need a dedicated team just to keep it running.
These platforms are great at what they do, but they're all missing something crucial: true autonomy. While your analysts are building dashboards about last quarter's performance, next quarter's challenges are already taking shape. While your data scientists are training models, market conditions are shifting. We're entering an era where manual analysis - no matter how sophisticated - simply can't keep up with the pace of business.
The Rise of Autonomous Business Intelligence
So what exactly is this new wave of analytics process automation? Whether you call it autonomous analytics, proactive analytics, or agentic analytics, the core idea is the same: moving from tools that help humans analyze data to intelligent systems that actively drive business outcomes.
Key Components of Analytics Process Automation:
Business Process Digital Twins
Digital twins are already having their moment in manufacturing and IoT, but their real potential lies in modeling entire business operations. Imagine having a living, breathing replica of your organization that captures not just what's happening, but why it's happening and what's likely to happen next.
These aren't just static models - they're dynamic systems that evolve with your business. When your market shifts, your digital twin adapts. When new data comes in, it updates its understanding. The magic happens when these twins start surfacing insights humans might miss: the subtle connection between employee onboarding times and customer satisfaction scores, or how slight variations in supplier delivery patterns ripple through to end-of-quarter revenue.
Context-Driven Intelligence
Analytics tell you what happened and where you are right now. AI-powered analytics tells you what's going to happen - and more importantly, what you should do about it. But unlike the black-box AI we've grown wary of, these systems are built for transparency.
The key differentiator is context. These systems don't just spot patterns - they understand your business objectives and frame everything through that lens. When they flag an opportunity or risk, they explain their reasoning in business terms, not technical jargon. "We're seeing a 23% uptick in customer support tickets" becomes "Based on current trends, we're projecting a $2M revenue impact next quarter unless we address the recent UI changes that are confusing enterprise customers."
Cross-Process Analytics Orchestration (All Of The Very Messy Kitchen Sinks)
Most enterprises are sitting on goldmines of data, but it's trapped in departmental silos. Cross-process orchestration breaks down these barriers, but it goes way beyond simple data integration.
The real value comes from understanding how everything connects. Maybe your HR recruiting metrics are secretly early indicators of product development velocity. Perhaps customer service chat logs contain insights that could transform your marketing messaging. These cross-functional insights often represent the biggest opportunities - but they're impossible to spot when everyone's working in their own data bubble.
The beauty of modern orchestration is that it's not just connecting data - it's creating a unified business narrative. It shows you how a small change in one area creates ripple effects across the organization, helping you optimize for global outcomes rather than local wins.
Real-World Applications
This isn't theoretical - autonomous analytics is already transforming how leading companies operate. Let's look at how this plays out in the real world:
Financial Services
Imagine a bank shifting from reactive fraud investigation to proactive prevention. Instead of teams spending their days investigating yesterday's losses, autonomous analytics could spot emerging fraud patterns in real-time. The system would identify suspicious patterns before money leaves the building, letting fraud teams focus on strategy instead of constant firefighting. No more morning meetings about what went wrong yesterday - instead, discussions about strengthening defenses for tomorrow.
Manufacturing Logistics
Automotive supply chains are highly complex. Traditional analytics might show you on-time delivery rates for each supplier. But autonomous analytics could discover how slight variations in delivery patterns create ripple effects that impact final product quality. By understanding these subtle interconnections, manufacturers could restructure their supply chains to optimize not just for timing, but for overall quality and efficiency. Instead of dealing with issues at final assembly, they could prevent them from happening in the first place.
Retail
The traditional approach to retail optimization is painfully slow: run a promotion, wait a month, analyze results, repeat. Autonomous analytics could transform this into a continuous optimization process across thousands of SKUs in real-time. Instead of making educated guesses about pricing or following competitors, retailers could let autonomous systems optimize prices based on real-time market conditions, inventory levels, and customer behavior. In an industry where a few percentage points in margin make the difference between success and failure, this could be game-changing.
The Bottom Line
Traditional enterprise analytics platforms are great at what they do. If you need heavy-duty data integration, Palantir's your go-to. Want to build predictive analytics models? DataRobot's got you covered. Need business intelligence dashboards? Tableau and Power BI are solid choices.
But if you're looking to actually transform how your organization uses data - to move from reactive reporting to proactive analytics - you need to be looking at autonomous analytics platforms.
The future isn't about building better tools for humans to analyze data - it's about creating intelligent systems that do the heavy lifting for us.
The question isn't whether autonomous business intelligence will become the standard - it's just a matter of when. The real question is: who's going to get there first?
Want to learn more about how AI-powered analytics automation can transform your organization? Get in touch to see Perceptura in action.